As a teacher, finding innovative ways to review crucial concepts with your students can be a game-changer, especially when it comes to complex science topics. Integrating fun classroom review games into your teaching strategy can break the monotony of traditional teaching methods in an interactive and collaborative learning environment.
I want to share with you five science-themed games for your next review sessions. Each game is designed to cater to various aspects of the science curriculum, making difficult topics more accessible and enjoyable for your …
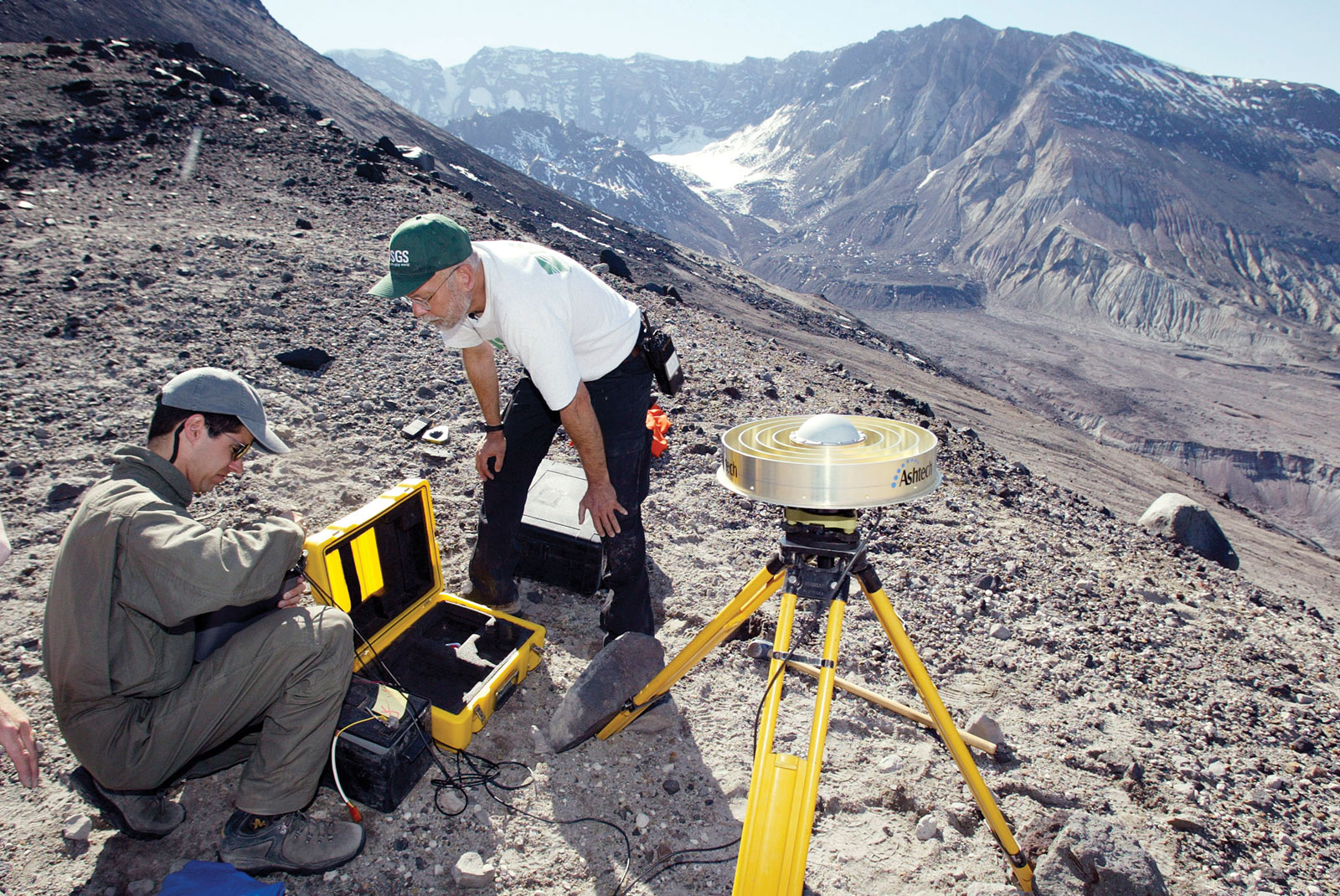
About Us
Science & Technology Research News features up-to-date news and discoveries by researchers and renowned scientists. The research work is done in top prestigious research universities in several countries, including the UK, Canada, the United States, Australia, and Asia. Since its establishment, this non-profit site is solely supported by the company’s partner universities from around the globe. Science & Technology Research News primary objective is to share the latest research news directly to the avid general population. We ensure that you are updated with news on Science and Technology….
Read MoreRenewable News
What are the safest and cleanest sources of energy?
25 people would die prematurely every year
people would die prematurely every year
people would die prematurely every year
In an average year nobody would die
Latest Earth News
What Does Simmering Water Look Like? A Complete Guide
Simmering is a fundamental cooking technique that falls between a gentle bubbling and a rolling boil. Understanding what does simmering water looks like is crucial for preparing a wide range of dishes and incredibly delicate foods.
Unlike the vigorous activity in boiling water, simmering is a more subdued and controlled cooking method. This technique benefits recipes with slow and gentle heat, such as soups, stews, and sauces. It allows flavors to meld and ingredients to cook thoroughly without the harshness of boiling, which can…
How to Fix Solar Lights
You can fix solar lights by replacing their batteries, cleaning their solar panels, replacing or reconnecting their cables, or replacing their sensors. But before you try fixing your solar lights, you have to figure out why it isn’t working.
Solar lights stop working for various reasons, including dirty solar panels, faulty light sensors, water ingress, and faulty connections. Whatever the case may be, you must detect the cause before trying to make your solar lights work.
10 Common Reasons Why Solar Lights Stop Working and …
Your Guide on Cleaning Solar Lights
With time, the solar lights installed in your home will require maintenance. These maintenance activities include cleaning the lights, replacing corrosive batteries, and restoring the panels to look as good as new. Cleaning your solar lights is very important if you want to keep them functioning optimally. Thankfully, solar systems are low-maintenance, but they still require your little input now and then.
Before diving into the methods mentioned above, you should know what to do before cleaning your solar system.
Pre-Cleaning Tips for your Solar Lights
Ensure you carry out the following before you put your cleaning …
Power Provider in Germany Tests Vertical Agrivoltaic Systems
Standing tall at 3 meters, two Bifacial solar modules are situated in Bavaria, Germany, right beside inveterate solar parks in Gersthofen and Biessenhofen. These two are small vertical agrivoltaic systems with 3 and 6 kW outputs to be tested by a power provider based in Augsburg called Lechwerke (LEW).
Lechwerke aims to obtain experience working with agrivoltaic systems with these pilot projects, a combination of plant production and photovoltaics, which has been brought to light as a synergistic synthesis of food production and renewable energy, then compare its performance with the existing ground-mounted projects.
The data…
How Solar Energy Optics Are Increasing Efficiency
Solar energy optics is a film solution made by the ICS. It results from a thorough industrial study focusing on light guide technology. A commercial solar panel can convert 15 to 20% of light energy, penetrating it into electricity. This is a notable improvement from only 12% a decade ago.
Nevertheless, if solar technology has better efficiency, it can contribute more to preventing climate change and developing the economy, specifically installations on rooftops.
Recently, some the companies such as Oxford PV, Insolight, and ICS have made a considerable improvements in the industry creating new advancements in improving solar…
How Pickling Can Lock Carbon in Oxygen-Free Ocean Depths
Did you know that there are parts of the ocean contain little to no oxygen? Due to this, there are no plants or aquatic animals that can live there. However, this type of environment is preferable to some microbes, and those are one of the places wherein they strive.
Because of climate change, these unique ecosystems continue to expand and strive. This is something that concerns fisheries and other locations that are dependent on seas rich in oxygen for livelihood and other aquatic activities.
But what piques the interest of a noted biogeochemist, Morgan Raven, is the continuous change of the chemicals in the sea and how it …
The Tropical Cyclone Yasa on Fiji
Fiji has been preparing itself for the inevitable arrival of a severe tropical cyclone known as Yasa. Citizens in the low lying areas have been issued warnings and advised to evacuate to escape the perils of the approaching storm. According to forecasters, the height of the waves in the eye of the storm could reach at most 45 feet in length or 14 meters. The eye of the storm appears to be moving straight ahead to the islands of Vanua Levu and VitiLevu.
Yasa emerged on December 12 as a tropical storm and had developed into a cyclone by December 14. The storm has had a lot of opportunities to gain momentum and growth. This is because …
The Effect of Climate Change in Oregon’s Western Cascades
A study claims that the changes in temperature and humidity due to climate change can lead to longer fire happenings and more brutal fire weather in the mountains of Oregon’s Western Cascades.
The lead author of the study, Andy Mcevoy, says that the changes in climate can lead to many possible scenarios that could lead to extreme fire situations. The study also suggests that there will be several days the components that create fire will align and cause fire happenings.
According to their simulations, there is a significant increase in the possibility of fire to happen in as little as eight days, with the peak number of…